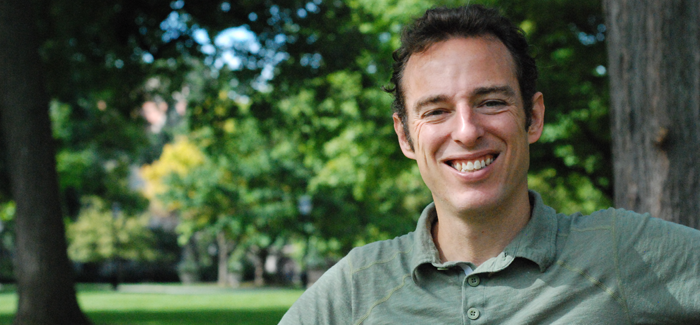
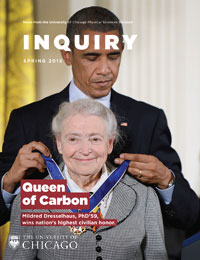
This article originally appeared in the Summer 2015 issue of Inquiry, the biannual publication produced for University of Chicago Physical Sciences Division alumni and friends.
In October Matthew Stephens, professor of human genetics and statistics, was named one of 14 investigators nationwide in the Gordon and Betty Moore Foundation’s Data-Driven Discovery Initiative. Stephens, who applies computation and Bayesian statistics (which deals with conditional probability) to population genetics research, will use the $1.5 million five-year unrestricted grant to study genetic variation and strengthen statistical methodology by improving the way methods are compared.
Describe the field of population genetics.
Population genetics studies genetic variation in “unrelated” individuals as distinct from studying genetic variation in families or related individuals. The interesting thing about unrelated individuals is that they’re actually all related, if you go far enough back. The part of population genetics that I’m interested in is how this distant relatedness affects the patterns of genetic variation we see in a population. Most genetic variants have arisen just once in the history of human evolution. If you share a genetic variant that I have, it’s usually because we inherited it from a common ancestor.
What will your genetics research focus on for the Data-Driven Discovery Initiative?
We’re trying to understand the molecular mechanisms underlying gene regulation, to identify the genetic variants that are affecting what’s going on inside a cell. Ultimately we’d like to understand how genetic variants impact the whole organism, but if we can start by understanding how they affect the cell, that’s a first step.
If a genetic variant is correlated with something, there’s a good chance that it could be causing the change. If X and Y are correlated, you don’t know if X is causing Y, Y is causing X, or neither. But we know that most genetic variants are fixed at birth, and they don’t change. We don’t have to worry about reverse causality.
Why does research reproducibility matter?
The way people conduct their research can have a big impact on how effective it is. One of the buzzwords in science right now is reproducibility. I’m interested in computational reproducibility, which means simply being able to reproduce your analysis, starting with the data, the code that you ran, and the output of the results. In principle that’s not as hard as one lab running an experiment and having another lab obtain the same result; you would think a computer is a controlled environment. But if you have any experience with computers, you’ll realize it’s not as controlled as you think. It requires incredible discipline for researchers to truly document everything they did in a reproducible way. It means automated workflow and never editing files by hand; a lot of people don’t have the computer tools.
Reproducing someone’s analysis is usually the first step to taking the next step, building on it, improving it, extending it—a way toward more efficient progress. I’m focusing on comparison of different statistical methods for different problems. Most people will write a paper but not publish the code they used. If they did publish that code and in a standardized framework, other researchers could add a method or a data set, and we could build up repositories of these comparisons.
Can you illustrate how a statistical method is tested and how comparing methods leads to a better outcome?
The usual way of testing a method is to use what’s called a training set of data, where you see both the predictors and the outcome and use those to learn about the relationship between the two. Then you give the program predictors; you know the outcome, but it doesn’t. It has to predict. An example of this system is movie recommendations. Netflix held a public competition to improve its recommendation algorithm. They used data from user-rated movies, and some of the ratings were presented and others were missing. For the purposes of the competition, Netflix held back ratings to assess whether the method made accurate predictions. There are different methods for doing that kind of thing, and people are developing new ones all the time.
Because the repositories will be open source, and some data—particularly genetic data—may be sensitive, how might you avoid problems with privacy?
There are at least two ways: you have to apply for access, or you have a third party run the programs on sensitive data sets. But there are all sorts of barriers to achieving that in practice. The best chance for a workable solution is for us to become more comfortable sharing genetic data. When I want to be controversial I tell people that in 10 years everyone will have their genomes on Facebook.